Here's an extra (Mal)teaser: what have chocolate bars got to do with data quality?
Think about a factory line making your favourite chocolate bar. Quality assurance is a key part of every step. If the product is faulty then no one will eat it, and the company wouldn’t make any money.
They know they have to invest to save. Not only in the machines on the production line, but in the raw ingredients too. They could have the most reliable, efficient and best factory machines, but if the raw ingredients had bits of hair or sand, or lumps of unmixed fat in, then they would waste money because they’d have a terrible product at the end. The machines help, but you have to make sure the quality of your raw ingredients is right.
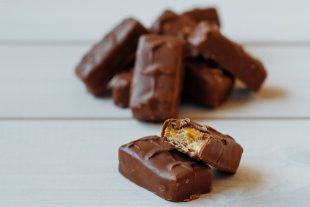
So, what’s the link be-Twix-t chocolate bars and data quality?
Replace the ‘factory machines’ with IT systems and the ‘raw ingredients’ with data. If I can stretch the analogy a little further, reactive data fixing is like paying someone to pick the bits out of the finished chocolate bars!
You wouldn’t eat a poor quality chocolate bar…
...so why accept poor quality data? We all consume data. It fuels our understanding of the environment and the decisions we make. Data is vital; we use it to allocate resource, make regulatory decisions and environmental improvements and to look after our staff to name just a few uses. Chocolate is a luxury but data is not! Approaching data quality in the right way matters.
As in a chocolate factory, we need clarity about the quality of the product we are producing and build in checks along the way to make sure we’re on track. If we don’t check our data we won’t know the quality and we won’t know if it’s wrong until it’s too late.
When errors are discovered we often default to cleansing errors in an ad hoc and resource intensive way. Low and behold, the quality (and fitness for purpose) of the data begins to drop again straight after the ‘fixes’ are applied. We don’t get to the root cause of the problem. Research from the wider data community suggests, on average, organisations waste 15–18% of their overall budget dealing with data issues. That’s a huge amount of wasted money and effort.
The manufacturing world wouldn’t accept such waste, so why accept it with our data?
Our challenge is to change our approach to data quality from reactive fixing to proactive changes.
As we publish more of the information we hold as Open Data we must become comfortable with others using our data. Some of our datasets are better than others, in quality of data and in quality of the processes that support them. We may worry about the quality of the data and others using it without understanding the detail of it. As we share it more widely, we shouldn’t Wispa it: our customers need to understand our data’s strengths and limitations, so that they can make better use of it.
What can you do?
If you look after data: It needn’t be a ‘rocky road’. Our approach to proactively monitoring data quality, called Data Quality Action Plans (DQAP), are based on what you need the data to do. They help lead identify the root causes of your data quality issues and gives you an evidence base to support putting effective long lasting fixes in place.
If you don’t look after data directly: We all make and use data, whether it’s environmental data, time recording or expenses. We are all responsible for its quality. Remember there’s no substitute for getting it right first time and we all have a responsibility to input accurate data. If you have a concern about the data you are inputting or using, raise it. Y'or-kie* to saving time and effort in the long run!
*You are key. It's stretching it a bit, admittedly.
3 comments
Comment by GDD posted on
Great article!
I'm just glad you resisted the urge to talk about the amount of rubbish people consume and the amount they push out! The quality of data has a direct impact on the helath of an organisation and its ability to grow. Unfortunately, some organisations just don't know how ill they really are or how obsese they've become and how much it is slowing them down.
Comment by Matt posted on
Of course data will not always match up with the aspirational requirments. Better to properly capture the conditions the data was captured under and report that along with the data itself. I can cope with uncertainty and error, as long as I know that it exists. Too often data is presented without this kind of metadata and creates a false sense of security.
Comment by Allan McBain posted on
As RPA's Quality Assurance Manager I feel compelled to comment. Then again I also feel compelled to have a piece of that Rocky Road my sister made and brought me yesterday.
...........
OK, back to commenting again, no pause there at all, honest.
Actually all I'd add to a great article is that the owners of the data have to consider the users as their customers and when users raise issues, take those on board so that we can create continuous improvement cycles - indeed the customer feedback on how data is performing should be like gold dust to the data owners. I am not, of course, accusing data owners of not considering it so, just the article only covered rasing issues.